What is Customer Churn?
As highlighted, customer churn is when a brand loses its customers due to several reasons. The causes of customer churn can be bad product features, poor customer experience, lack of engagement, pricing, competitive market dynamics, etc.
Some of these issues can be from the brand’s side, and some customers may leave just because they want to. I need to tell you that some volume of customer churn is necessary.
Pro tip: As per stats, a churn rate of 4% is considered good. Moreover, the average churn rate of a business annually is 5-7%.
Based on the causes of customer churn can be of several types, including
- Voluntary churn
Just like its name, voluntary churn is when a customer actively decides to stop using the products and services of a brand. As teased above, there are several reasons for voluntary churn, such as bad product features, poor customer experience, better options in the market, etc.
- Involuntary churn
Unlike voluntary churn, involuntary churn is not due to customer preferences. It is due to expired credit cards, payment issues, outdated payment information, or server errors. The brand or business has to take action to ensure that the customer does not face these errors.
They can do this by upgrading or fixing their payment servers, informing the customers regarding their credit card issues, etc.
- Active churn
This is when the user actively cancels his or her subscription. In this process, the customer follows a formal cancellation process, informing the business about their decision.
- Passive churn
In this, churn happens because the user does not actively inform the brand about leaving or canceling the subscription. They just don’t renew their subscription, leading to a higher churn risk.
What is Churn Prediction, and Why is it Important?
Customer churn prediction is a method by which businesses tend to find out about the customers who are at a high risk of churning. There are several ways to do it, such as studying the user dataset and online activities. The customer churn prediction process also informs the reasons for churn.
As there are a number of parameters required to predict customer churn, a churn prediction model is imperative. A customer churn prediction model can also help you find the most loyal customers for the brand.
Besides this, there are a plethora of other reasons to go for churn prediction methodologies!
- Boost customer retention
Customer retention can be way more cost-effective than customer acquisition. With a customer churn prediction model, it is easier to identify the important aspects of your product that might be causing customers to churn based on NPS, CSAT, rage clicks, etc.
Based on the analysis of these aspects, required product changes can be done made faster and the users at the risk of churn can be retained.
Do you know!
Customer acquisition can cost you five times more than retaining one. Moreover, the success rate of selling to a new customer is 5-20%, whereas it is 60-70% to an existing customer.
- Build customer persona
Customer personas can be of great use if you know how to crack them. Customer personas are categories into which you divide your customers based on certain characteristics. For example, if you have a product with features X and Y, 10 people will like feature X, and 20 people will like feature Y.
You can make two customer personas here and further align your goals to reduce churn by focusing on improving the features in the corresponding cases. User personas can help you understand specific pain points and target them with solutions for maximum product sales.
An example of this can be Swiggy! The Indian food delivery brand builds user personas to target them with healthy meals and snack options. In this image, a customer persona is shown with all the necessary details.
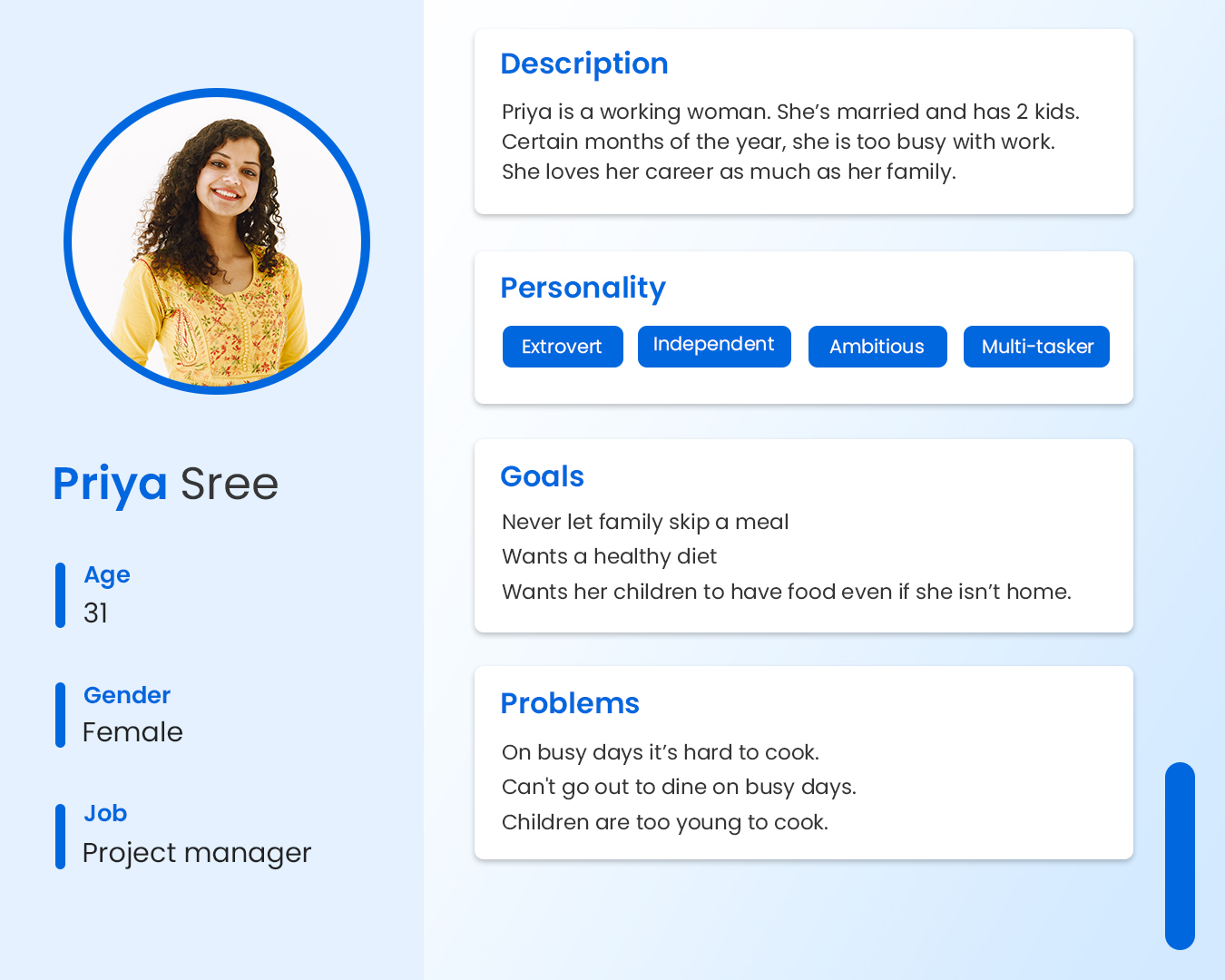
You can check out some more examples here!
- Make re-engagement campaigns
With churn prediction, you are more likely to know about customers who are about to churn. With this knowledge in mind, you can easily create re-engagement campaigns for them. The re-engagement campaign aims to target those customers who have been inactive for some time period.
This is to ensure that these inactive customers re-engage with the brand. These re-engagement campaigns include monetary and non-monetary incentives to trigger customer purchases or subscriptions.
An excellent example of this is Grubhub!
Grubhub is an American food delivery company that applied an awesome re-engagement strategy to target customers who have their app but haven’t placed any orders till now
The brand provided a flat discount of $10 for an order over $15.

- Make focused customer success content.
Several customer data points can help you predict churn; once you know the cause, it becomes easier to craft a solution. For example, there can be some apps or products that users may find difficult to use.
In such a case, focused and helpful content can be created to help them and further retain them to reduce churn. This content can be digital manuals, in-app tutorials, etc. There are many apps on the market that help the user get used to it by providing a tutorial.
I remember when I started playing the game Hill Climb Racing, the game began with providing a tutorial about how to accelerate the vehicle, apply brakes, perform a wheelie, and more. These small features in the product can help in keeping the customer churn rate low.
Here are some of the effective ways to manage churn!
What is a Churn Prediction Model?
A churn prediction model is a method to predict if the customer will churn or not based on the historical data of the customer and powerful supervised machine learning algorithms. The process includes several comprehensive steps.
The process begins with the capturing user data. The key thing to ensure here is that you should have a parameter to track in order to run the churn model.
Furthermore, you need to have multiple predictive aspects or parameters based on which you will know if the customer churned or not. These parameters can be the type of subscription, cost of the subscription, usage data, etc. Based on the comprehensive analysis of these parameters, you can predict churn.
Now, let’s come to the analysis part! The data acquired regarding customer behavior has to be analyzed using machine learning models. For predicting churn, there are several machine learning techniques that you can leverage. These innovative techniques can help identify patterns in the data and make predictions for customer churn.
Here are some of the machine learning techniques that I find useful to predict churn before it happens.
Well, this is an overview of the churn prediction model. However, when you have to build a churn prediction model using machine learning and data science, certain aspects play a crucial role.
How to Build A Customer Churn Prediction Model to Predict and Prevent Churn?
I need to highlight one thing here. Data is everything in a churn model. If you want to know whether a customer is likely to churn, you need to focus primarily on data preparation. Here is how you can do it.
- Data preparation for churn analysis
The data preparation stage includes a number of aspects, such as multiple data points related to the existing customers. These can be feature usage data, customer behavior data, clicks, time to value, interactions, etc.
Further, you can obtain more data from customers through NPS and CSAT surveys. Besides these, you can conduct other surveys for more real-time data, like an in-app survey.
Once all of this data is in your hands, it is time to clean the data to remove any missing values or irregularities. It can be done via several methods, such as normalization, outlier detection, etc.
- Exploratory Data Analysis
Once the data is cleaned, the next step to build a model to predict customer attrition is data analysis. Begin with analyzing customer data to predict the reasons behind churn. These can be high prices, bad customer experience, invaluable products, etc.
Based on these factors, you can perform further in-depth analysis of data. There are two ways to conduct data analysis here: manual data analysis or an automated predictive model.
Both of these models will help you correlate customer aspects with churn risk. For example, in the manual method, you can compare the app usage with the churn rate of the customers. You can also use NPS vs churn to estimate if a low NPS score is leading to churn.
An automated predictive model handles churn prediction using machine learning. These methods are a bit complex, but they deliver the best and most accurate results.
- Customer churn prediction and prevention
Based on the manual and automated data analysis methods, you can predict churn and prevent it from happening. There are several methods to improve customer retention while preventing churn, such as providing incentives, seamless app onboarding, interactive walkthroughs, educational material, etc.
These ways can also help bring back churned customers if they are re-engaged in a planned manner.
Essential Software Features for Predict Churn!
I know that you must have mastered the ways to create a churn prediction model. However, you will still need a powerful tool to perform all your data analysis and visualization. I suggest Qwary as the best tool for this.
I have used the tool, and I have seen several utilitarian features for easy churn prediction. Here are some of the best features that I like about Qwary!
- User insights
User insights can include a number of aspects, including app usage time, demographics, customer satisfaction over time, rage clicks, and whatnot. The collective analysis of all these aspects helps in analyzing user behavior and further taking steps to reduce customer churn.
Qwary offers session recordings, in-product surveys, AI analysis, heatmaps, etc. features that can help in decoding user insights for you.
- Dashboards
Dashboards are a great resource of Qwary where you can easily visualize all the necessary data elements like overall NPS, CSAT, customer feedback sentiments, session recording analytics, etc.
Moreover, the organic view of the dashboards ensures easy data analysis for faster action management.
- Customer Journey Logs
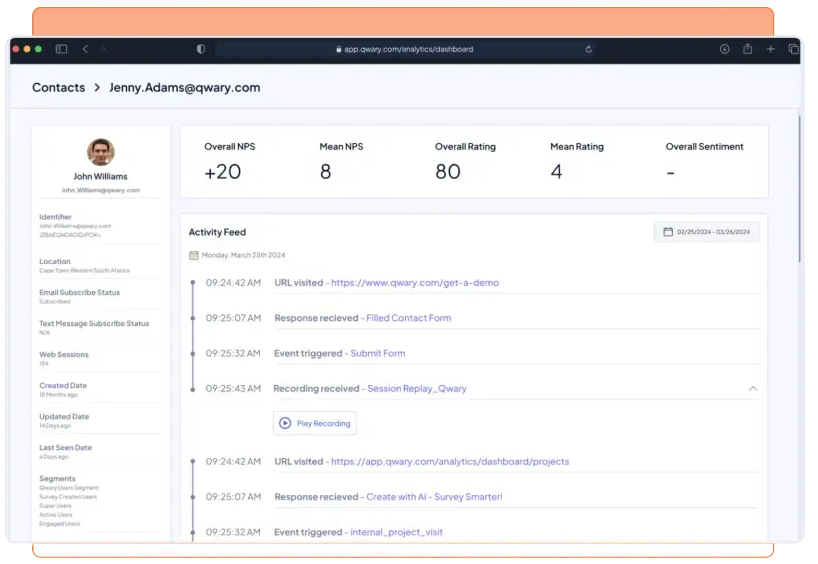
Customer journey is an essential feature of Qwary and it is the most important from the business perspective. With an insight into user journey logs, you can find out which pages the user visited, what actions they took, how far they researched the website, user sessions, and whatnot.
Based on all this information, you can analyze which part of your website is favoring conversions and which isn’t. Furthermore, you can make smart churn prevention decisions, such as optimizing the specific pages of the website.
I believe that if you are a small business yet, you should focus on every aspect behind every customer using your product. It will be time-consuming, but it will highlight every issue in your website’s user experience.
Besides these, several other features of Qwary can come in handy for accurate churn prediction, such as heatmaps, trend analysis, CX analysis, AI analysis, etc.
Conclusion
In the end, I want to add that a churn model is indispensable to find customers who are likely to churn. But, it has to be planned well as data is the critical element in it. Data has to be collected from all the sources and cleaned thoroughly to get accurate results. If you are a large business dealing with heaps of data, it is crucial to focus on data pre-processing, as a single mistake can cost you a lot.
If I talk about the best tool for you, it is, without a doubt, Qwary, which can help you unravel cutting-edge insights for accurate churn prediction. This can help in boosting overall customer retention, customer loyalty, and conversion rate.
FAQs
- What is customer churn prediction?
Customer churn prediction is a process of detecting which of the customers are more likely to churn. It is necessary to have a churn prediction model as it costs a lot more to acquire a new customer than retain one.